Artificial Intelligence vs Machine Learning: Exploring the Key Differences
• Customer Support
• FastBank
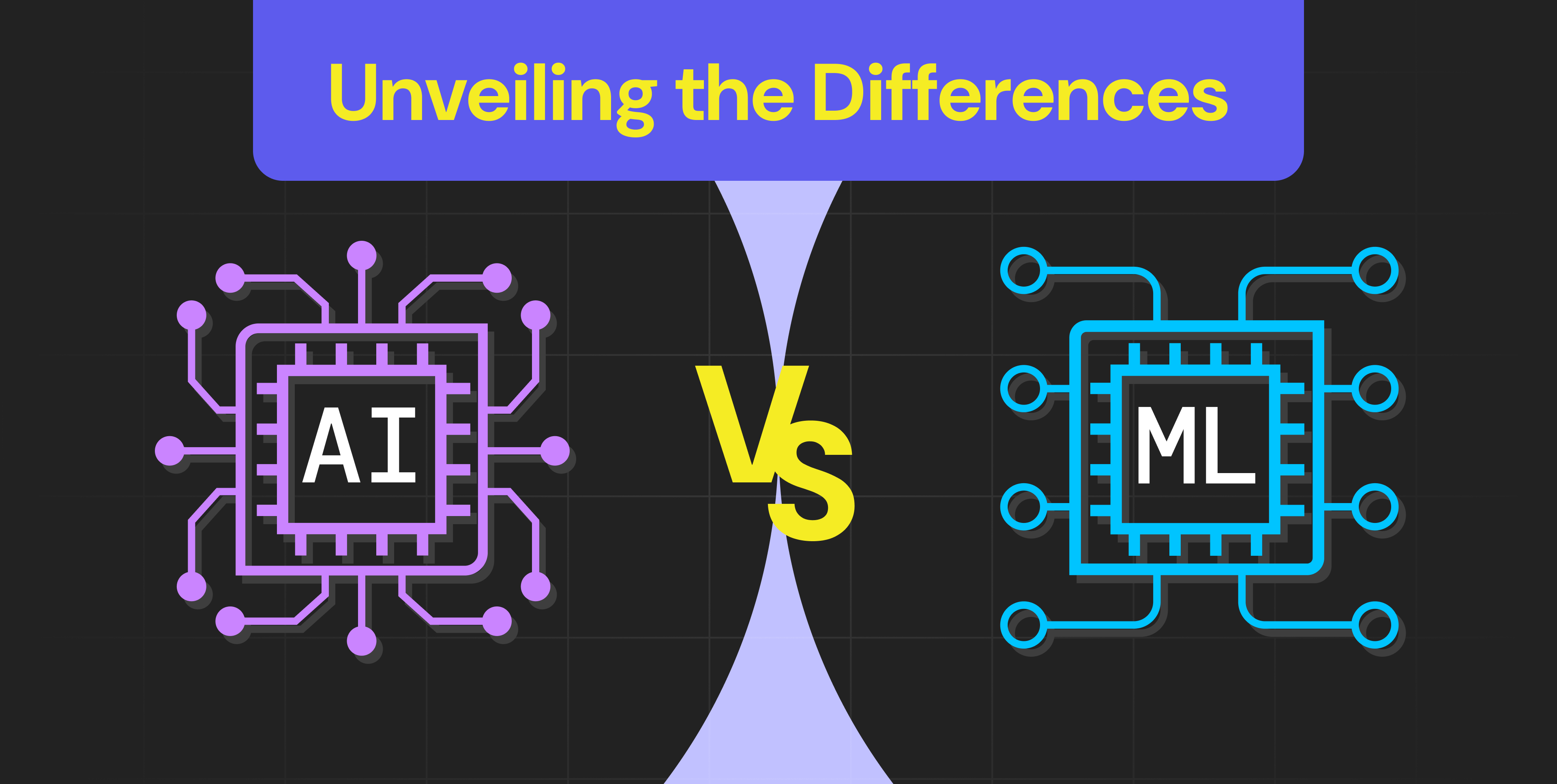
Imagine a world where robots make decisions on their own - learning from data rather than following predefined rules. This is the essence of the debate between artificial intelligence (AI) and machine learning (ML).
In the tech world, AI and ML are buzzwords that are often thrown around as if they were one and the same, but they each play unique roles in the development of smart technologies.
But these are different gears in the machinery of advanced computing. "Machine learning is the science of getting computers to act without being explicitly programmed," says Arthur Samuel. In contrast, AI encompasses a broader spectrum, including rule-based systems and decision-making algorithms.
According to a July 2024 study, the global AI market is valued at over $196 billion, highlighting the massive impact and investment in AI applications.
In this article, we'll discover the core differences between these two pillars of tech innovation, exploring how each contributes uniquely to the evolution of intelligent systems. Dive in to understand where machine learning ends and AI begins and how this distinction impacts future technology.
Understanding Artificial Intelligence (AI)
The term "artificial intelligence" was first used in a proposal in 1956 by John McCarthy, Marvin Minsky, Nathaniel Rochester, and Claude Shannon during a research conference. They wanted to explore how to program computers to mimic human brain functions. Later, McCarthy defined AI as the science of making smart machines, especially computer programs, that can achieve various goals.
There is no second opinion that AI has been reshaping our world at an unprecedented pace. As we go deeper into AI applications, it's crucial to clearly differentiate it from its counterpart, machine learning. In this section, we dive into AI to explain the key principles that power many of today's popular technologies and tools, ranging from AI virtual assistants to autonomous vehicles.
What is AI?
AI refers to the simulation of human intelligence in machines designed to think and act like humans. These intelligent machines are programmed to perform tasks that typically require human intelligence, such as visual perception, speech recognition, decision-making, and language translation. AI systems are designed to learn from experience, adapt to new inputs, and perform human-like tasks with a high degree of accuracy.
Understanding Machine Learning (ML)
In 1959, AI researcher Arthur Samuel introduced "machine learning." He trained a computer to play checkers better than most humans by having it play thousands of games and learn from each one. Samuel described machine learning as the ability of a program to improve its performance through experience.
What is ML?
Machine learning is a subset of AI that involves the development of algorithms and statistical models enabling computers to learn from and make decisions based on data. Unlike traditional programming, where explicit instructions dictate the system's actions, machine learning allows systems to identify patterns, make predictions, and improve over time with exposure to more data. Machine learning powers various applications, including recommendation systems, fraud detection, image and speech recognition, and autonomous driving, making it a critical component of modern AI technology.
AI vs ML: Core Differences
AI and ML are two of the most talked-about technologies today, driving innovation across numerous industries and fundamentally altering how we solve problems and interact with technology. While they share similarities and are often mentioned together, they serve different purposes and have unique characteristics.
Figuratively said, AI is like the big dream of making machines smart enough to do things that usually need human brain power. It's all about creating systems that can understand, reason, and interact like people do. Imagine AI as the overall goal of creating super-smart robots that can handle any task a human can.
ML is a piece of that big dream. It's more about teaching these machines to get better at specific tasks by learning from data. Instead of being programmed with rules for everything, ML systems learn from patterns in data and get smarter over time.
So, AI is the big picture of making machines smart, while ML is the way we teach them to improve and adapt through experience.
Here's a practical breakdown of their core differences:
-
Scope and Definition
AI encompasses the ambitious goal of replicating human intelligence in machines. This involves a broad spectrum of technologies, such as natural language processing, computer vision, robotics, and advanced decision-making algorithms. AI is the grand vision of creating machines that can perform any intellectual task that a human can and sometimes even surpass human capabilities.
ML, a subset of AI, focuses specifically on developing algorithms and models to process data and make predictions or decisions based on that data. The ultimate goal is to enable systems to improve their accuracy and performance over time through iterative learning. Unlike AI’s broader scope, ML is about designing methods for systems to automatically learn and adapt from data without being explicitly programmed for each new task.
-
Application
AI has a broad and varied application range, touching many sectors:
Healthcare: AI applications include diagnostic tools that assist doctors in detecting diseases earlier, personalized treatment plans tailored to individual patients, and robotic surgery systems that enhance precision.
Finance: AI is used in algorithmic trading to analyze market trends and execute trades at optimal times, fraud detection systems to identify unusual transaction patterns, and risk management tools.
Customer Service: Intelligent chatbots and virtual assistants use AI to handle customer inquiries, while sentiment analysis tools measure customer satisfaction and feedback.
Entertainment: AI drives content creation by generating personalized recommendations, enhancing user experiences in gaming with more adaptive and intelligent Non-Player Characters (NPCs), and enabling new forms of interactive media.
ML is particularly valuable in areas where analyzing data patterns is crucial:
Recommendation Engines: ML algorithms analyze user behavior and preferences to suggest products, movies, or services tailored to individual tastes.
Image and Speech Recognition: ML models are used to identify objects in images, transcribe spoken words into text, and understand natural language commands.
Predictive Analytics: ML techniques forecast future trends based on historical data, such as predicting customer churn, sales forecasting, and detecting anomalies.
Future Trends in AI and ML
Now that we've explored the key differences between AI and ML, it's time to shift our focus to the horizon of technological advancements. In this section, we'll delve into some of the most noteworthy future trends in AI and ML. Let's go!
-
AI-Based Cybersecurity: AI-based cybersecurity uses artificial intelligence to enhance the detection and prevention of cyber threats. AI systems can analyze vast amounts of data to identify patterns and anomalies indicative of malicious activities, allowing faster and more accurate threat detection. These systems can also adapt and learn from new threats, continuously improving their defense mechanisms. This proactive approach helps protect sensitive information and critical infrastructure from increasingly sophisticated cyber attacks.
-
Self-Supervised Learning: Self-supervised learning is a machine learning approach where models learn from unlabeled data by creating their own supervision signals. This method enables models to harness large amounts of data without the need for extensive human annotation, making the learning process more efficient and scalable. This approach significantly reduces the dependency on labeled datasets, accelerating advancements in various AI applications.
-
Federated Learning: Federated learning is a decentralized machine learning technique where models are trained collaboratively across multiple devices or servers without sharing raw data. Each participant trains the model locally on their own data and only shares model updates, preserving data privacy and security. Federated learning is particularly useful in sensitive domains like healthcare and finance, where data privacy is crucial.
-
Edge ML: Edge ML refers to deploying machine learning models directly on edge devices, such as smartphones, IoT devices, and edge servers, rather than in centralized cloud servers. This approach reduces latency, increases processing speed, and enhances data privacy by keeping data processing local. By distributing computational tasks to the edge, this trend also alleviates network congestion and reduces dependency on constant internet connectivity.
Last Words
To conclude, machine learning and artificial intelligence play distinct yet complementary roles in advancing technology. Machine learning focuses on enabling systems to learn and adapt from data, while AI encompasses a broader range of techniques aimed at emulating human-like decision-making. By understanding these roles and how they intersect, we can leverage their combined potential to drive smarter solutions and groundbreaking advancements.
At Hoory AI, we bring this intersection to life with our advanced AI-powered customer support automation tool. Experience firsthand how our solutions integrate AI and ML to transform customer service. Try Hoory AI today and see how it can revolutionize your approach to customer support.